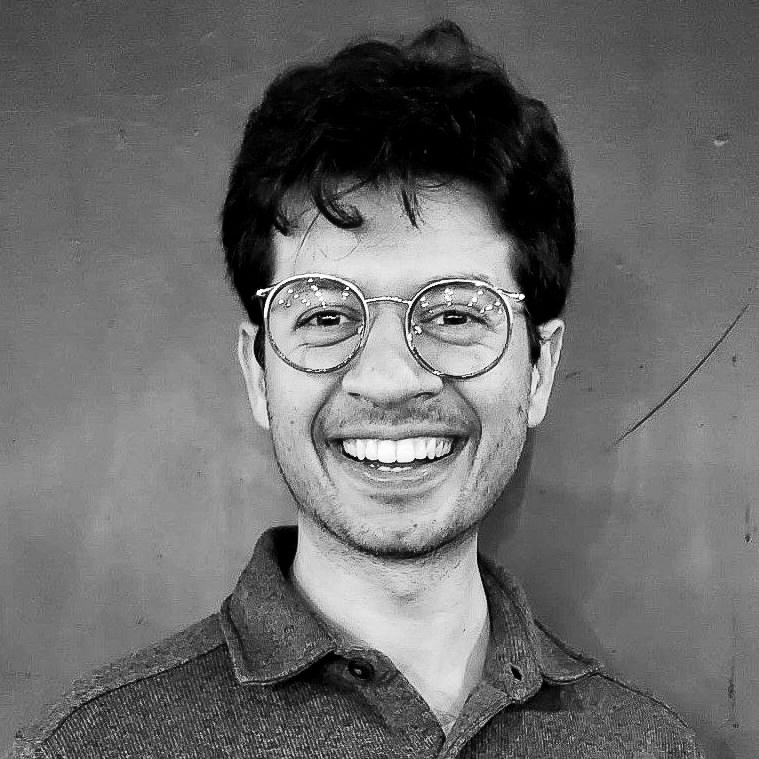
Gagan Bansal
Senior Researcher, Microsoft Research
Bio
Gagan Bansal (he/him) is a researcher at Microsoft Research, Redmond where he conducts interdisciplinary research on Artificial Intelligence and Human-Computer Interaction. His research broadly focuses on enabling human-AI interactions that help augment human performance. This involves evaluating, understanding, and improving these interactions via better interfaces or models. Initially, his work was focused on interaction with classifiers, however, recently, his focus has shifted towards interaction with generative AI, specifically Large Language Models (LLMs) and LLM-based agents. He played key roles in creation and maintenance of AutoGen, a widely used open-source framework for developing multi-agent applications, and AutoGen Studio, a popular low-code interface for authoring multi-agent workflows.
At Microsoft Research, he is part of the Human-AI eXperiences Team and AI Frontiers. Prior to joining MSR in 2022, he graduated with a Ph.D in Computer Science from University of Washington, Seattle where he was advised by Dan Weld and was a part of the UW Lab for Human-AI Interaction. While working on his Ph.D, he did two research internships at Microsoft Research with Besmira Nushi, Ece Kamar, and Eric Horvitz. Prior to joining UW in 2015, he completed his B.Tech in Computer Science from IIT Delhi where he worked with Mausam on Natural Language Processing.
Checkout select projects and publications below.
Select Publications
Students
I have had the opportunity to advise incredibly talented students during my time at UW and Microsoft.
Interns at Microsoft
- Omar Shaikh (Stanford, Summer 2024, Co-advised with Adam Fourney and Eric Horvitz)
- Grace Proebsting (Haverford College, Summer 2024, Co-advised with Adam Fourney)
- Will Epperson (CMU, Summer 2024, Co-advised with HAX)
- Enhao Zhang (UW, Summer 2024, Co-advised with Eric Zhu)
- Shannon Shen (MIT, Summer 2023, Co-advised with Adam Fourney)
- Martin Josifoski (EPFL, Summer 2023, Co-advised with Adam Fourney and Eric Horvitz)
- Valerie Chen (CMU, Summer 2022, Co-advised with Jenn Worthman Vaughan and Vera Liao)
- Helena Vasconcelos (Stanford, Summer 2022, Co-advised with Adam, Jenn, and Vera)
- Hussein Mozannar (MIT, Summer 2022, Co-advised with Adam Fourney and Eric Horvitz)
Students at UW
- Cindy Su (Co-advised with Ben Lee, Summer 2021-Fall 2021)
- Prithvi Tarale (Autumn 2020-Summer 2021)
- Joyce Zhou (Co-advised with Dan Weld, Autumn 2019-Summer 2020)
- Jacob Sippy (Co-advised with Dan Weld, Autumn 2018-Summer 2020)
- Lynsey Liu (Co-advised with Jonathan Bragg, Autumn 2017-2018)
- Ziyao Huang (Co-advised with Jonathan Bragg, Autumn 2017-2018)
- Diana Iftimie (Co-advised with Dan Weld, Winter 2017)